Overview
To answer the research questions AI4NetMon will be based on a data-driven approach, leveraging on rich heterogeneous network data and state-of-the-art ML/AI methods.
The project will develop a set of open-source tools, aimed to be used by Internet operators, researchers, and practitioners (without requiring expertise in ML/AI). The AI4NetMon tools will receive as input the available network data and provide as output answers to the key questions (e.g., potential locations for new monitor deployments, monitoring selection strategies, unbiased estimates for a set of measurements).
Specifically the project objectives are to:
Identify the key use cases for monitoring infrastructureWhich are the best locations to deploy monitors? What is the best set of probes to conduct measurements from? ...There is not a single answer to these questions! The best measurement strategy depends on what one wants to measure; e.g., a best set of monitors for detecting an outage, may be different than the best set of monitors for estimating an anycast catchment. Since a global answer does not exist, we need to provide answers per case. AI4NetMon will identify the use cases of higher importance to network operators, and for which the monitoring infrastructure is more frequently used.
Quantify the bias and efficiency of the existing infrastructure and potential for improvementWhat is the accuracy, coverage, and bias of the existing monitoring infrastructure (for a certain use case)? What would be the best achievable performance? Is there a margin for improvement? How much? Building upon preliminary findings (paper), AI4NetMon aims to answer these questions through theory, measurements, and experiments.
Develop open-source tools for efficient Internet monitoringAI4NetMon will develop AI methods and tools to (i) identify the best locations (e.g., ASes, IXPs, geographical regions) to deploy new infrastructure, (ii) select set of vantage points from the existing infrastructure for lightweight and efficient measurements (e.g., subset of Atlas probes or route collectors), and (iii) unbias estimations from existing measurements. The AI4NetMon tools will collect data from various heterogeneous data sources and aggregate them in a common dataframe, which will feed state-of-the-art AI that will provide recommendations, predictions, inferences, etc., for the desired monitoring objectives.
Project results
Bias: Quantification, Metrics, Results
We quantify the bias in the Internet measurement/monitoring infrastructure and platforms. We first define the bias and the metrics to quantify it. Then we compare the bias in the different Internet measurement infrastructures (e.g., RIPE Atlas, RIPE RIS, RouteViews) and present results that visualize this bias. The answers to the following questions
- How biased is the infrastructure? (e.g., from a scale from 0 to 1, where 0 is no bias)
- Is RIPE RIS more biased than RIPE Atlas? If yes, how much? What about RouteViews?
- Is the infrastructure more biased in terms of location or network sizes?
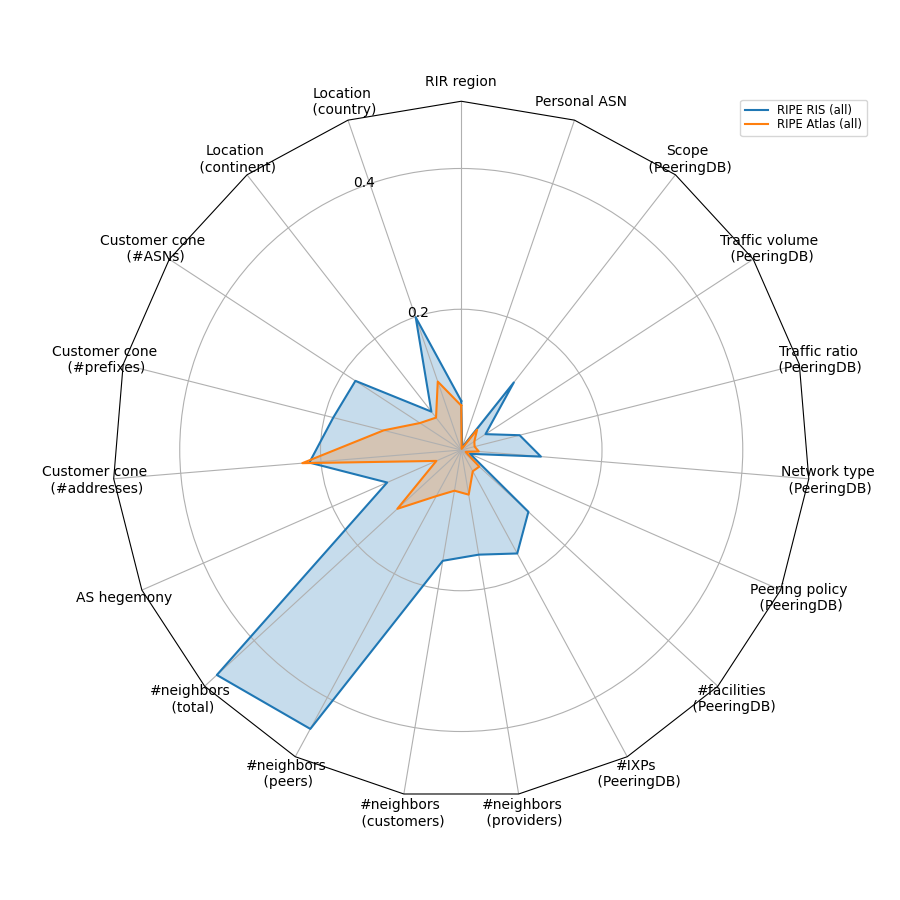
Recommendations for deployments of extra RIPE RIS and RIPE Atlas vantage points
We aim to find new potential locations for RIPE RIS monitors (i.e., ASes to peer with RIPE RIS). When adding an AS to the RIPE RIS monitors, then (i) the bias of the RIPE RIS changes (can increase or decrease), and (ii) there is some "improvement score" in terms of proximity to origin ASes, i.e., the total distance in AS hops to origin ASes decreases).
The following plot shows what is the trade-off by adding an AS to RIPE RIS: bias difference by adding this AS to RIPE RIS (x-axis; the smaller the better) vs. the ``improvement score'' it adds to RIPE RIS (y-axis; the larger the better).
For detailed description and results see our online tool.
A quick view for 5 recommendations for extending the RIPE RIS and Atlas infrastructures with vantage points in ASes that could decrease the bias. For more data, details, and options see the online tools for RIPE RIS and RIPE Atlas
Subsampling from Internet measurement platforms to decrease measurement bias
One can select subsets ("subsampling") of the vantage points of the Internet Measurement Platforms (IMPs), such as, RIPE Atlas, RIPE RIS, or RouteViews, in order to decrease their bias and get significantly more representative measurement data. In this documentation, we provide (i) detailed results for the bias of subsampling in IMPs, and (ii) a method for efficient subsampling.
The AI4NetMon API
We provide an open API, which provides the bias scores for different IMPs of custom sets of vantage points. The API can be accessed at https://ai4netmon.csd.auth.gr/api/, and at its endpoints are described in this documentation.
Articles / Blog posts / Presentations
-
"AI4NetMon Web App Tutorial" [Presentation], in RIPE NCC Open House Series, 27 September 2023. [ Link] [ Slides]
-
"Bias Causes in Internet Measurement Platforms: the case of RIPE RIS" [Blog/Tool], in Observable notebook, 1 September 2023. [ Link]
-
"Bias in Internet Measurement Platforms" [Presentation], in IEEE/IFIP TMA conference, 29 June 2023. [ Slides]
-
"Bias vs. volume of information" [Blog/Tool], in Observable notebook, 18 May 2023. [ Link]
-
"AI4NetMon - Analysis for MANRS members" [Blog/Tool], in AI4NetMon web, 13 February 2023. [ Link]
-
"Bias in Internet Measurement Platforms" [Blog/Tool], in Observable notebook, 27 October 2022. [ Link]
-
"Bias in RIPE RIS per Route Collector" [Blog/Tool], in Observable notebook, 27 October 2022. [ Link]
-
"Extending RIPE Atlas in an unbiased way" [Blog/Tool], in Observable notebook, 17 August 2022. [ Link]
-
"Extending RIPE RIS in an unbiased way" [Blog/Tool], in Observable notebook, 29 June 2022. [ Link]
-
"Revealing Bias in Internet Measurements" [Podcast], in RIPE Labs, 29 Jun 2022. [ Link]
-
"Bias in Internet Measurement Infrastructure" [Presentation], in RIPE84, 17 May 2022. [ Link]
-
"Bias in Internet Measurement Infrastructure" [Blog], in RIPE Labs, 28 March 2022. [ Link]
-
"Potential deployments of extra RIPE RIS monitors: Bias vs. Improvement" [Blog/Tool], in Observable notebook, 14 February 2022. [ Link]
-
"Estimating the Impact of a Hijack: Measurement Bias and How to Avoid It" [Presentation], in RIPE83 (Routing WG), 22 November 2021. [ Link]
-
"RACI Funding in 2021 - Planning and Projects" [Blog], in RIPE Labs, 17 November 2021. [ Link]
Publications
[C3]
Pavlos Sermpezis, Lars Prehn, Sofia Kostoglou, Marcel Flores, Athena Vakali, Emile Aben
"Bias in Internet Measurement Platforms",
in IEEE/IFIP TMA conference, 2023.
[ PDF]
[ slides]
[ Code ]
[C2]
Dimitrios P. Giakatos, Sofia Kostoglou, Pavlos Sermpezis, Athena Vakali
"Benchmarking Graph Neural Networks for Internet Routing Data",
in ACM CoNEXT - GNNet workshop, 2022.
[ PDF]
[ Code ]
[C1]
Pavlos Sermpezis, Vasileios Kotronis, Konstantinos Arakadakis, Athena Vakali
"Estimating the Impact of BGP Prefix Hijacking",
in IFIP Networking conference, 2021.
[ PDF]
[ Presentation ]
[ Code ]
Code
-
AI4NetMon [ github repo ]
-
Analysis of RIPE Atlas usage patterns [ github repo ]
People
Principal Investigator
Pavlos Sermpezis, Datalab, AUThResearch Assistants
Sofia Kostoglou, Datalab, AUTh
Theodoros Diamantidis, Datalab, AUTh
Stelios Mantzouranis, Datalab, AUTh Christos Georgitsis, Datalab, AUThResearchers / External Collaborators
Emile Aben, RIPE NCC
Lars Prehn, Max Planck InstituteWeb Developer
Stelios Karamanidis, Datalab, AUThAdvisor
Athena Vakali, Datalab, AUTh
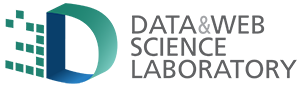
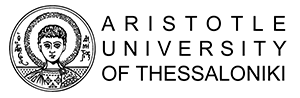
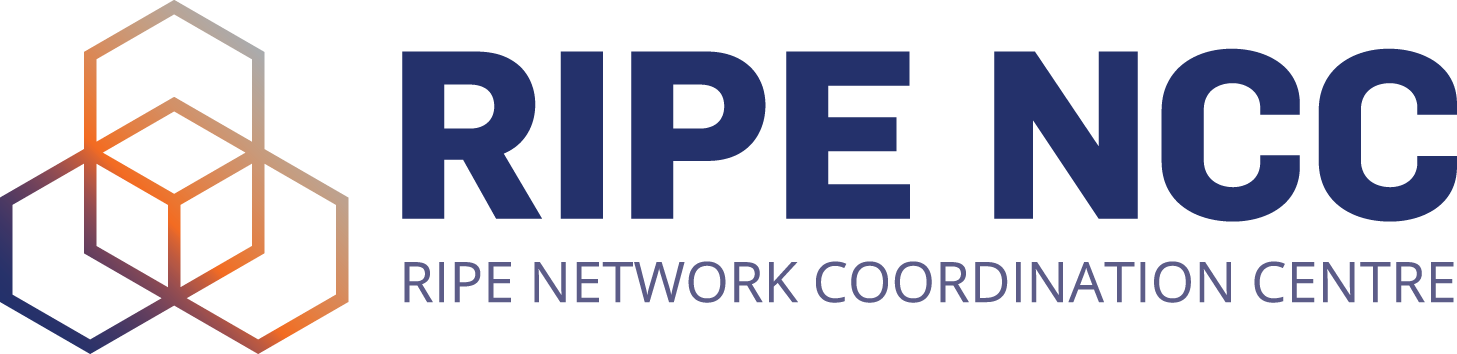
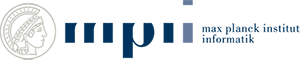
Contact
Funding/Acknowledgements
AI4NetMon is funded by RIPE NCC, through the RACI project funding 2021 and the RIPE NCC Community Projects Fund 2022 programs.
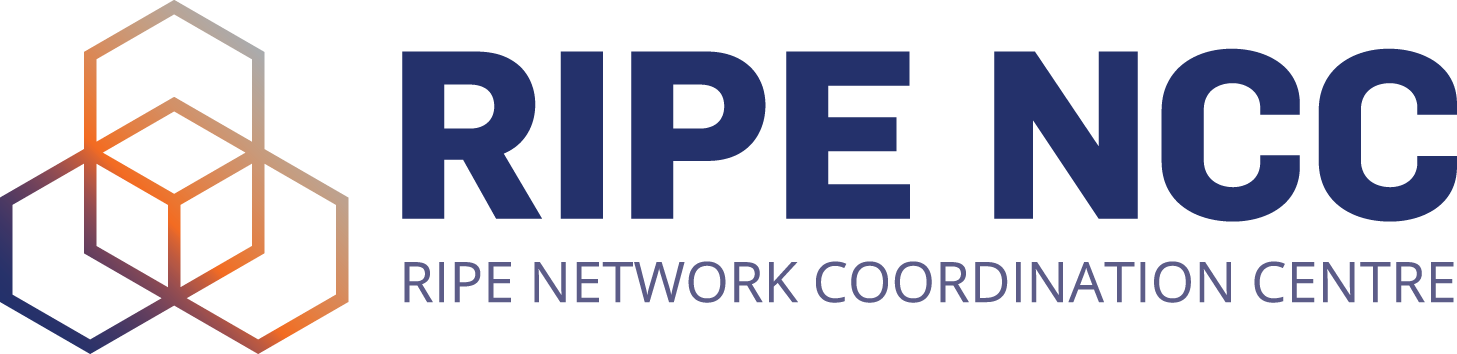